A look inside NeuroBlu: Structured socioenvironmental stressor data
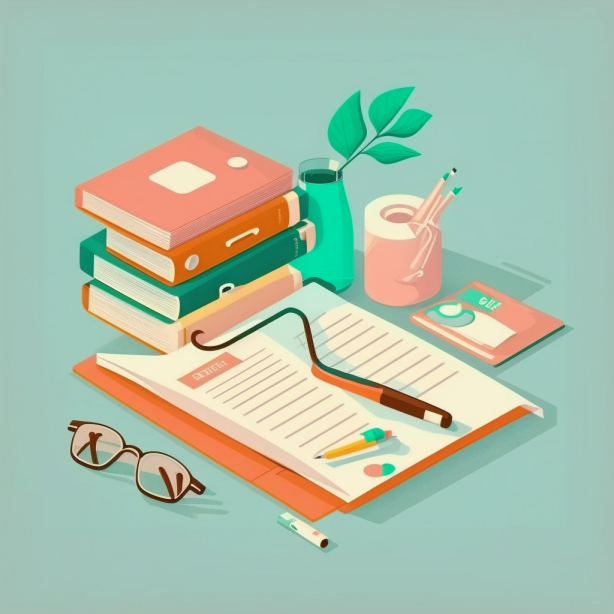
Huge amounts of data are collected throughout a person’s journey through the behavioral healthcare system. From demographic data captured during intake to in-depth conversations between patients and clinicians about symptoms, daily activities, and goals, most of the information captured about a patient becomes part of their electronic health record (EHR).
As Holmusk’s VP of Clinical Informatics and Analytics, I think about the different pieces of information contained within the EHR and how we can pull these pieces together to accurately portray a patient’s journey and ultimately, to use this information for research. We are continually thinking through which data are needed to answer the most challenging behavioral health questions, and how to ensure these data are available for easy access through our NeuroBlu data analytics software. As we continue to pay special attention to the environment’s impact on mental health during Mental Health Month, I wanted to provide a brief overview of some of the socioenvironmental stressors that can currently be analyzed using NeuroBlu.
Demographics
Even through the most basic demographics collection, we still obtain data on socioenvironmental stressors patients may be experiencing. For example, a patient’s zip code can tell us which community they live in, which is an important indicator of health outcomes. Early in my career, I worked for an organization in Northern Virginia where we noticed a drop in program participation and an increase in no-shows at a particular location. After continued data analysis and speaking with front-line clinicians, we discovered a bus line had been discontinued. This lack of transportation was a socioenvironmental stressor that significantly impacted our patients’ ability to access much needed safety net mental health services. A shuttle service between locations was quickly put into place and after a few weeks, program participation increased and no-shows decreased.
In addition to the zip code, NeuroBlu contains other socioenvironmental demographic data such as age, ethnicity, gender, race and city/ state of residence.
Housing data
Many of our data partners, whose data is added to the NeuroBlu Database, collect information on whether a patient is homeless or in a suboptimal living situation. Clinicians will often document housing status and circumstances surrounding their living situation in a structured and unstructured manner. Structured data related to housing that are available within NeuroBlu include data related to stress and abuse at home, problems living alone, homelessness, inadequate and unstable housing. All of these data points contribute to understanding the patient’s journey and what may be contributing to their mental illness.
Housing instability can contribute to anxiety and other mental health symptoms; conversely, having a serious mental illness can impact a patient’s ability to obtain housing. Many behavioral health organizations have independent housing programs to provide this basic human need while the patient stabilizes on medications and learns activities of daily living skills. However, this housing is funded on a temporary basis, and many clinical programs struggle with discharging individuals into permanent affordable housing.
Adults Needs & Strengths Assessment (ANSA)
We are adding different types of data constantly as our NeuroBlu Database rapidly expands. Our latest release, which became available last week, includes the Adult Needs & Strengths Assessment (ANSA). This scale has a lot of interesting information on a patient’s specific strengths in different domains, including spiritual and religious connections and community connectedness. As is the case with most of the scales contained with our NeuroBlu Database, the ANSA includes question-level data, making it easier to pinpoint individual strengths, as well as socioenvironmental stressors that may be inhibiting these strengths.
Daily Living Activities
Another assessment that is captured within the NeuroBlu Database is the Daily Living Activities Functional Assessment. This scale uses clinician observations and direct questioning of the patient to make conclusions about their functioning. Domains covered by this scale range from personal grooming to relationships with family, giving good insight into socioenvironmental stressors the patient may be experiencing.
Pros of observations and assessments
The NeuroBlu Database contains a wide variety of assessments, and because the data is longitudinal, each assessment is likely to be captured multiple times for the same patient. This enables easy tracking of patterns and can help providers identify when patients are improving or declining. One example of this is the ANSA assessment mentioned above. In many U.S. states, the ANSA and CANS (Child and Adolescent Needs and Strengths) assessments are required as part of the pre-authorization and payment for behavioral health services rendered. The assessments are required every 6 months, which provides a holistic look into the patient’s functioning throughout the course of treatment.
Cons of observations and assessments
The same observations and assessments are not collected at each clinic throughout the country, which sometimes makes direct comparison difficult when conducting research. This can be attributed to a number of factors, including variable insurance requirements that mandate different assessments to be administered in different states, as well as a lack of provider bandwidth. Often, providers have to choose between administering an assessment or actually treating the patient, and because their time with each patient is limited, they are likely to choose the latter. This heterogeneity in collection of scales and assessments leads to some limitations around structured data, which we supplement with unstructured data, as my colleague Abhijit will expand upon in next week’s blog.
Look Around, Look Within
Our data partners have already begun the important work of helping their patients to “look around, look within,” and have recorded telling data about their environments. Our work at Holmusk centers on ensuring these data are available and easily interpretable for a wide variety of behavioral health stakeholders, from providers to life sciences researchers.
Although we already have much valuable information available, and are adding more structured scales and assessments all the time, we have barely scratched the surface of all the data we could ingest into the database, data that would help paint an even more complete picture of a patient’s environment. Most of this data sits within unstructured fields in the EHR and has not historically been leveraged for deeper learning. Stay tuned for next week’s blog for a continuation of our recognition of “Look Around, Look Within”—and a look into how we are capitalizing on the untapped potential of unstructured data.