The value of NeuroBlu Data: Reflections on our study in The Lancet Psychiatry
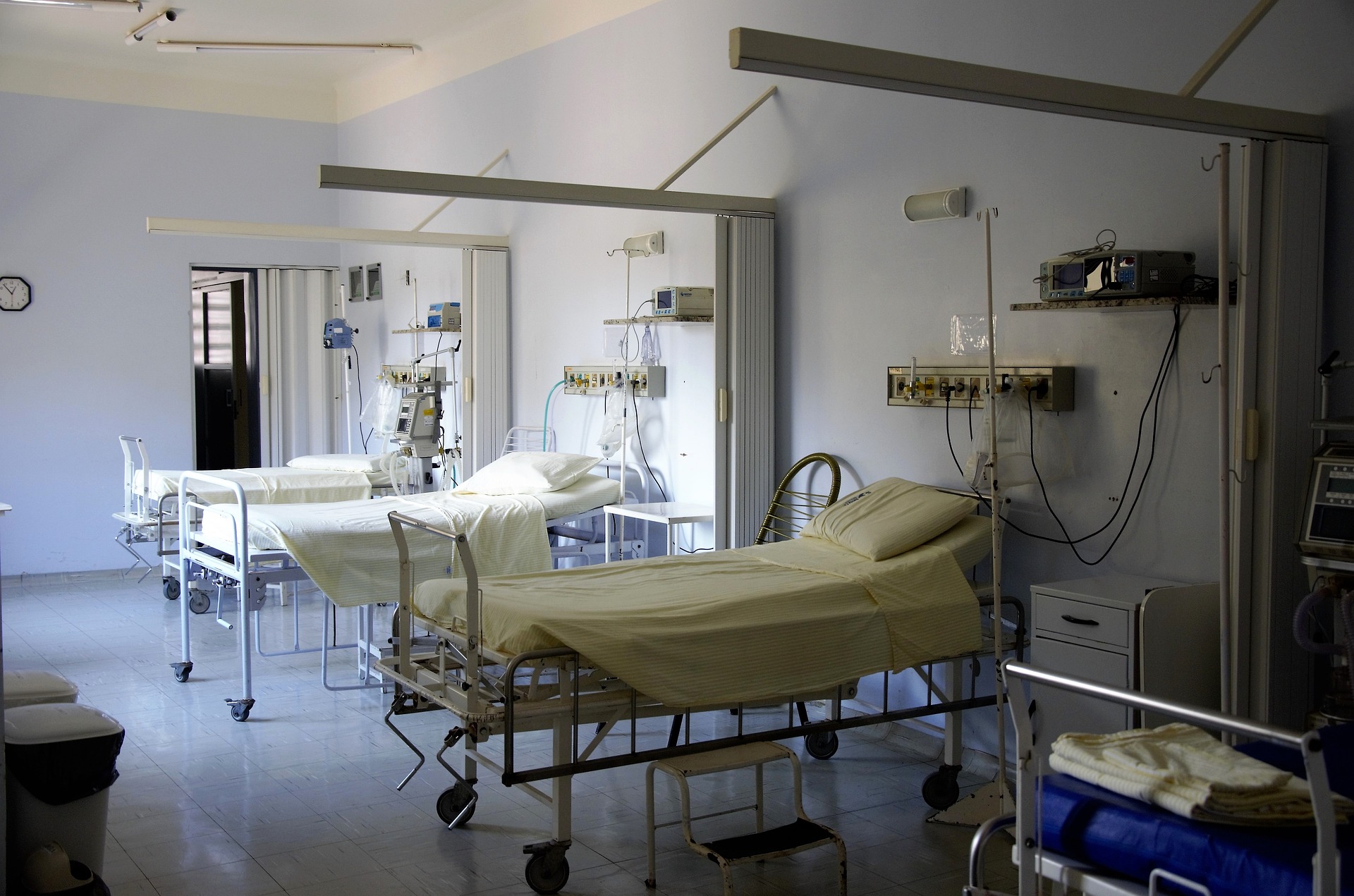
I recently led a team of researchers in a large-scale observational study, which was accepted and published by The Lancet Psychiatry. To our knowledge, our study was the first to study the impact of early clinical trajectory across multiple psychiatric diagnoses.
The study’s findings—which you can read in full in the journal—were interesting. Most notably, those patients who scored in the top half for clinical severity and instability were at a 45% higher risk of psychiatric hospitalization within the next six months. And perhaps even more interestingly, this association remained consistent across diagnosis, age, or sex.
Because this study did successfully include multiple diagnoses, I wanted to reflect on a few of the key features of the data source that fueled our research: the NeuroBlu Database.
Breadth & depth
When I initially was introduced to the NeuroBlu Database, I was impressed by not only the size of the dataset—the continuously growing database now contains de-identified records from over 1.4 million patients—but also by the types of data that were available within this EHR-derived real-world data. Knowing that this unique combination of breadth and depth was the only way to address challenging research questions in behavioral health, I agreed to collaborate with Holmusk on a consultancy during which I would use their NeuroBlu data to conduct research.
Collection of scales
The NeuroBlu Database also features frequent recording of standardized measures such as psychometric scales that are collected by clinicians during routine care. From the 1.4 million patients, we were able to pull a sizable study cohort of over 36,000 patients receiving care for behavioral health conditions, who had each received frequent assessments of their illness severity using the same scale.
We initially used the Clinical Global Impression Severity (CGI-S) scale to measure illness severity and instability. In a field like behavioral health, which has traditionally been challenged by a lack of standardized measurement, these scales are invaluable in facilitating research by enabling us to make easy comparisons across patients.
And because the NeuroBlu Database contains so many different scales, we were able to repeat our original analysis to test for robustness, this time swapping in the Patient Health Questionnaire (PHQ-9) for the CGI-S. Findings from this analysis complemented our original results—which was very interesting because the first analysis used a scale administered by a clinician, while the latter used a patient-reported outcome measure.
Longitudinal data
Because the NeuroBlu Database contains longitudinal data spanning 20 years, it was easy for us to follow patients for six months after they initiated care to identify which patients were hospitalized during the six-month period. If real-world data had been collected at only one point in time and was not longitudinal, this type of analysis would not have been possible.
Real-world data sources are so important in conducting research because they can present a clearer picture of how care is actually delivered. Not only does this give us a better understanding of patients’ lived experiences, but it also delivers results that are more directly translatable to clinical practice, which is critical to improving patients’ outcomes. The NeuroBlu Database offers a valuable treasure trove of this kind of information, with data on individual scales and symptoms that are captured widely across the U.S.