Data missingness or expectation misalignment? A look into a common critique of real-world data
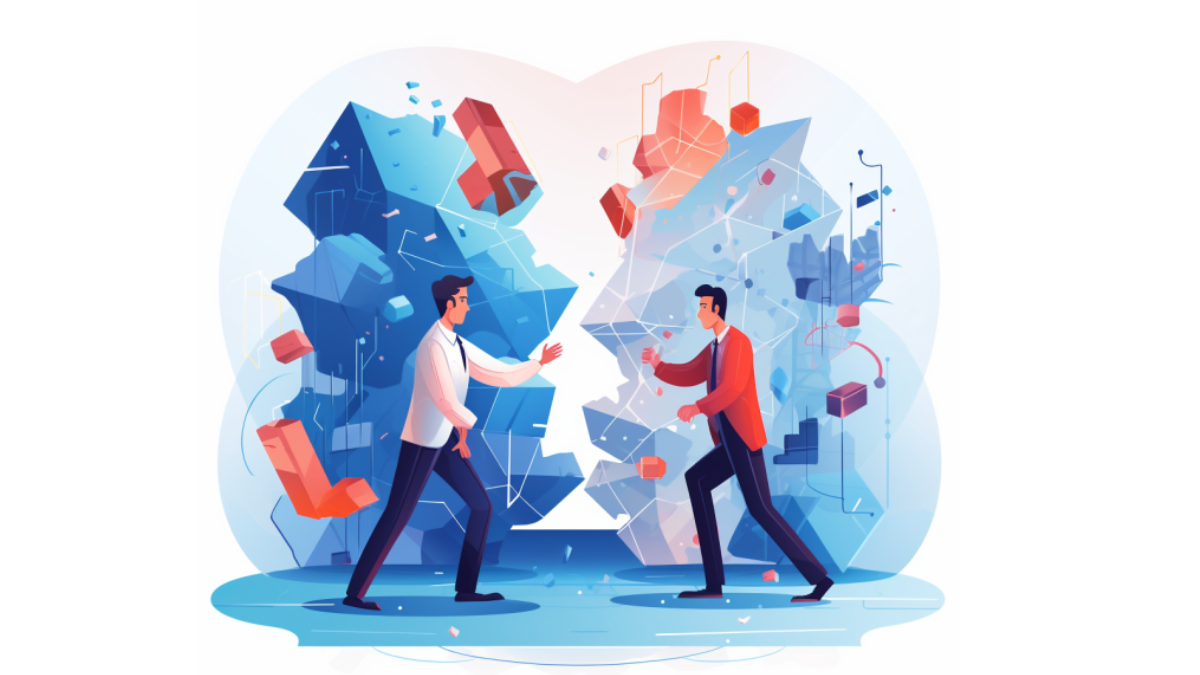
As biopharmaceutical companies and regulatory bodies look toward adopting EHR-derived real-world data to complete studies more efficiently and affordably, one major concern that often arises is what has come to be known as “data missingness.”
The theory is that completeness of real-world data is impacted by the wide variability in how data is collected in clinical practice. Health systems use different EHR systems, and there are differences in how individual providers may record information in the EHR, which is often dependent on the amount of time the provider has to capture data while simultaneously delivering care to the patient. Conversely, in the highly regulated, controlled environment of a clinical trial, data is captured on a rigorous, standardized schedule with little variation, which some believe leads to better “completeness” and less “missingness.”
But what if this theory of data missingness isn’t entirely sound? I’ve worked as both a mental health clinician and as a curator of clinical data from the EHR, and from both perspectives, I can tell you that data capture in the real world often is complete, with nothing “missing” at all.
Instead, what is happening is a misalignment—a mismatch between what is actually feasible during a real-world visit to the clinic and the expectations of those who are accustomed to highly standardized clinical trial data, such as those working in drug development or regulatory settings. In order to successfully move forward and realize the benefits that EHR data can add to research, including faster studies and lower costs, these stakeholders will need to understand that while the EHR and clinical trials are two separate settings that cannot always be directly compared, they will, more often than not, be able to address the same clinical questions.
Same answers, different methods
Historically, researchers have relied on certain measures often used in clinical trials to better understand aspects of a person’s condition, such as symptoms or severity. Although these measures provide valuable information, they are not inherently valuable because they take too long to capture in a routine appointment with a patient. This means that often, the conditions of patients who participate in clinical trials are measured and monitored completely differently than those of the typical patient receiving care in a real-world setting.
One example of this, which my colleague Alex touched on in a previous blog, is the usage of the Montgomery-Asberg Depression Rating Scale (MADRS) and the Hamilton Depression Rating Scale (HAM-D) versus the Patient Health Questionnaire (PHQ-9). Despite multiple studies that have shown the PHQ-9 is just as valid as the other two scales to measure depressive symptoms, clinical trials continue to use lengthy measurement scales that are infeasible to implement in clinical practice, furthering the disconnect between the study setting and the clinical setting.
This misalignment is seen across conditions, which often leaves the field with no standardized way to objectively measure symptoms within diagnoses. Another example is how the field identifies positive and negative symptoms of schizophrenia. Many clinical trials rely on the Positive and Negative Syndrome Scale (PANSS) or the combination of Scales for the Assessment of Positive Symptoms (SAPS) and Scale for the Assessment of Negative Symptoms (SANS) to measure these symptoms. If stakeholders working in drug development looked at a typical real-world dataset, they wouldn’t see these measures—because they are rarely collected in the real world—and may conclude the dataset suffers from data missingness. However, it is likely the dataset has measures that are used in the real world that measure the same symptoms adequately; in the case of identifying schizophrenia symptoms, the PSRS, BNSA, and BPRS show a lot of crossover with the traditionally accepted scales, while being much shorter and more feasible to implement in clinical practice.
Adjusting expectations will yield results
When specific measures are missing from real-world datasets, stakeholders must adjust their expectations of real-world data and learn how to use different types of data, such as measures that are given more frequently in practices, to provide the information they seek. Not only does the EHR contain the information that is needed, it also, in many cases, contains a deeper wealth of information than could ever be obtained from clinical trials, as real-world data includes patients typically excluded from clinical studies.
If stakeholders fail to align and adjust to readily available measures in the EHR and continue to extenuate the false story of data missingness, they will miss out on all of the benefits that EHR data provides—research with lower burden, easier cohort identification, and more affordable studies being just a few.