Enhancing Data Density in EHR Data for Behavioral and Mental Health Analysis: The 4 I's Approach
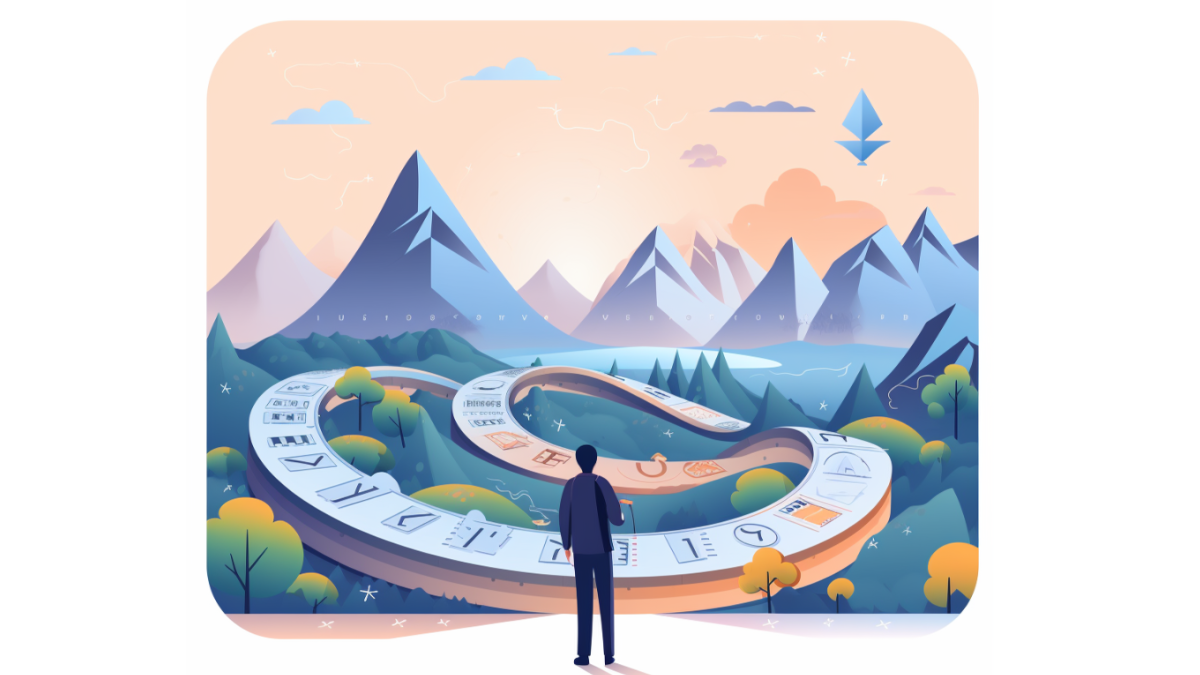
In the realm of behavioral and mental health, electronic health records (EHR) data is a goldmine of information. However, the challenge lies in the fact that these data often need more density for comprehensive analysis.
In a previous blog post, my colleague and Holmusk's Senior Vice President of Data Strategy and Operations, Alex Vance, gave a brief overview of the efforts Holmusk is undertaking to add density to EHR-derived data and ensure its value for future research. In this blog, I outline a four-part framework we utilize as we navigate through EHR data and increase its density before making it available via our data analytics software, NeuroBlu.
Investigate
Investigation is the first step in our journey. This involves exploring raw data from our partners’ EHR systems to understand what data types we will need to harmonize and ingest into our NeuroBlu Database. This process is crucial in understanding the depth and breadth of the information we have at our disposal.
The standardization process is a stepwise approach that maps semi-structured data to structured data and structured data to standardized concepts. This transformation allows us to make sense of the data, prepare it for further analysis, and ensure it is fit-for-purpose. By doing so, we enhance the density of the data, making it more valuable for our studies.
Impute
Imputation is the process of gleaning additional information from readily available data. Many techniques can assist with this imputation; for example, linear-based scoring against regression modeling can facilitate the prediction of patient trajectories, enriching data by predicting values where values may not be present.
In the context of EHR data, natural language processing (NLP) also plays a pivotal role. NLP enables the extraction of meaningful information from unstructured text within EHRs. Within our data analytics software NeuroBlu, we have recently released NeuroBlu NLP, beginning with a depressive disorders NLP model that can scan clinical notes and predict the presence or absence of anhedonia and suicidality.
Additionally, we derive measurements through methods such as smoothening (which helps to interpolate values, identify patterns in data, and eliminate outliers), crosswalks (which enable direct comparison between different standardized measures), and identifying relationships between different types of structured data to impute measurable outcome data. These techniques allow us to create a more complete and accurate picture of a patient's health, thereby increasing the density of the data.
Integrate
Integration involves adding new data sources to support our analysis. Sometimes, we find “new” data by continuously working with our existing data sources to maximize the value derived from each source and ensure that as much existing data as possible can be integrated and made available via NeuroBlu. This process can result in discovering additional tables or measures that can be mapped to our common data model.
Integration can also include adding other real-world data types, such as claims data, patient-reported outcomes, and other relevant sources. In NeuroBlu, we’ve integrated data from ongoing and completed clinical trials in behavioral health. This facilitates comparison between clinical trial cohorts and real-world data cohorts with similar inclusion and exclusion criteria that can be created within NeuroBlu.
By integrating multiple data sources, we improve the density of our data, ensuring deeper insights to address behavioral health challenges.
Influence
Influence is the final step in our journey—perhaps the most difficult milestone. It involves influencing stakeholders across the behavioral health ecosystem to come together and implement changes that result in collecting denser data from the outset. Denser data will result in improved evidence, which will facilitate consensus-building when it comes to setting a standard of care. These multi-faceted efforts should include encouraging providers to take more frequent measurements while simultaneously persuading providers to provide the incentive and reimbursement structures necessary to make this increased measurement a reality. Other stakeholders, including life sciences companies who develop treatments and the regulatory arms that approve them, must also be involved to foster agreement on data collection standards and definition-setting.
These efforts, while challenging, ensure that we are not only leveraging the data that exists today but also working to shape the future of data collection in behavioral and mental health. By influencing these key stakeholders, we can continuously improve the level of data density in behavioral health studies.