How NLP-enriched data advances clinical research
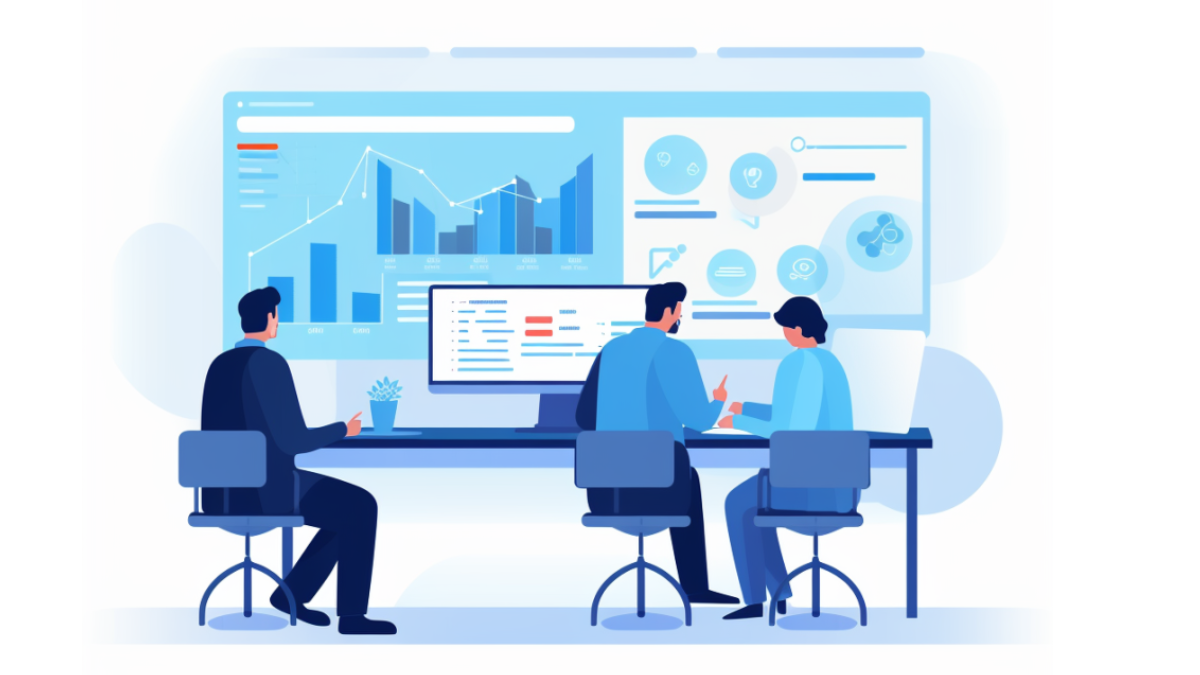
Real-world evidence has been increasingly recognized as an important complement to clinical research. In clinical research, randomized controlled trials often have stringent inclusion criteria which may exclude certain patient groups, such as those with comorbidities. When clinical trial populations diverge from patient populations seen in a real-world care setting, insights gained might not be widely applicable to all people. Analyzing real-world data, such as clinical information recorded in electronic health records (EHR) as part of routine clinical care, can provide insights that are generalizable to real-world populations.
However, all real-world data does not provide the same level of value. Studies that rely on the structured fields contained within EHR-derived datasets will face limitations in terms of the breadth and depth of data available. Structured EHR fields only capture some types of information, such as patient demographics, treatments and diagnosis. There are structured clinical rating scales which describe illness features such as patient symptom presentation or severity. However, these take a long time to perform and are therefore infrequently used in clinical practice and often missing in structured fields.
Holmusk moves beyond structured data fields, solving for their limitations by leveraging unstructured data such as clinical notes. Unstructured EHR data is a rich source of clinical information and can be used alongside structured data to offer deeper patient characterization. Holmusk analyzes these unstructured data within clinical notes in a scalable way by using natural language processing (NLP). NLP extracts key clinical features and transforms them into discrete variables that can be used for research. This blog highlights just a few of the ways that research can benefit from leveraging NLP-enriched data, such as the data made available via NeuroBlu NLP.
More granular patient phenotyping
Patient phenotyping is particularly challenging in the behavioral health field, as two people with the same diagnosis may experience different sets of symptoms and disease presentations. NLP-enriched data helps to solve for this by allowing researchers to access and aggregate information about clinical features and disease presentation in notes recorded by individual clinicians during clinical visits. At the 2022 Annual Congress of the Schizophrenia Internal Research Society (SIRS), we demonstrated that applying NLP to EHR data is an effective method to estimate positive and negative symptom burden in patients with schizophrenia and that a greater number of recorded positive or negative symptoms was associated with greater illness severity. With such dense data about each patient, it is easier to create a granular cohort of patients with similar features of research interest.
Clinical features which can be used as outcomes
The information extracted via NLP can not only be used in research studies to describe clinical features of patients but also their treatment or illness outcomes. For example, NLP can detect presence or absence of suicidality. This information could be used in a study that investigates features associated with mental health crisis as an outcome.
Better understanding of side effect burden
Holmusk is also working on NLP models that can extract information about side effects. This information, like symptoms, can also be used to characterize populations of interest. For example, a researcher could build a cohort of patients with schizophrenia who also experience tardive dyskinesia. An analysis we conducted and presented earlier this year at SIRS 2023 showed that using structured data to identify tardive dyskinesia (as defined by having the corresponding ICD-10 code in a patient’s record) is inadequate in capturing the true number of people who experience this side effect. Unstructured data can be used to account for similar gaps in official diagnosis.
Rapid, scalable, and cost-effective approach
NLP transforms unstructured notes into structured, and therefore analyzable, data. Previously, this information was accessible only through time-consuming, manual extraction that is nearly impossible to perform at scale. Although these same types of data can often be collected prospectively in a study, this would be quite costly, and studies often only capture the data that are relevant to their endpoints.
With NLP-enriched data, we can gain access to large cohorts with the potential for more comprehensive and precise phenotyping. Leveraging NLP can accelerate research and support the development of more targeted treatments—and we can do all of this at a faster pace and a lower cost than ever before.