Capitalizing on untapped value: Extracting environmental stressors from clinical notes via natural language processing
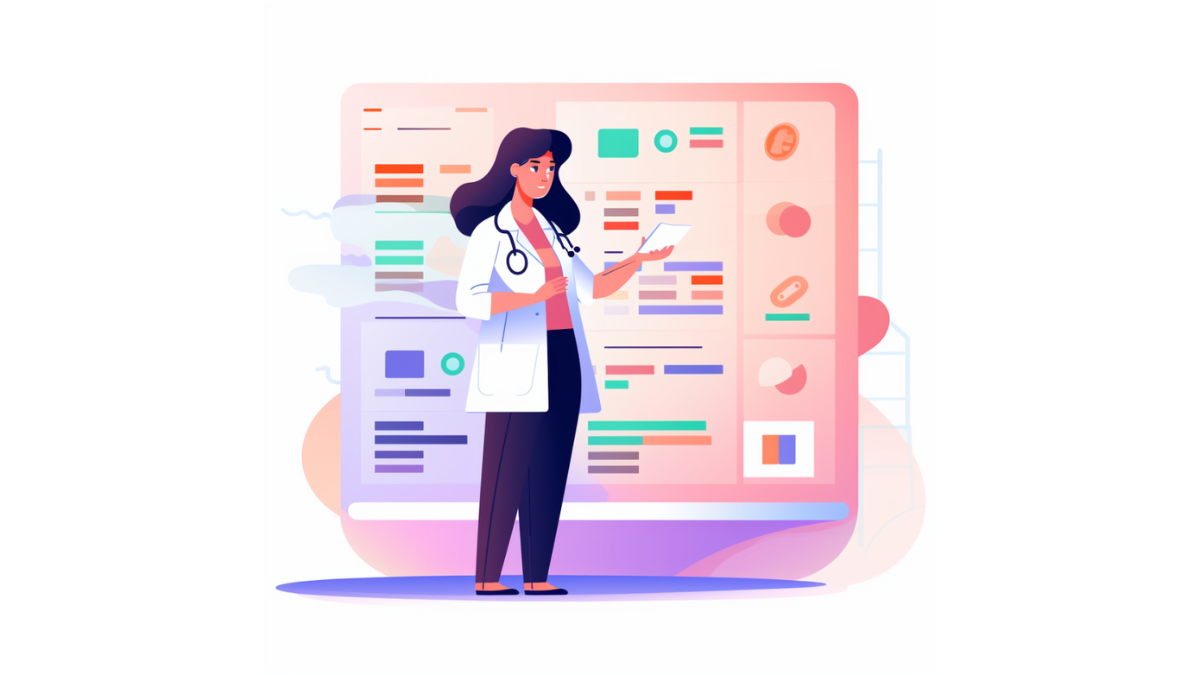
Consider an emergency room doctor who is caring for a patient who has been hospitalized after a suicide attempt. When she consults his chart, she can see that he has been diagnosed with depression, but the structured data that is immediately available does not provide much additional context.
However, if there was a way for her to easily access the information from the patient’s assessment notes–the unstructured data within a patient’s record that his therapist has recorded at each visit–she would be able to see that three months ago, her patient went through a divorce. Gaining this insight would enable her, and the rest of the patient’s care team, to provide proactive care and management that considered the socioenvironmental stressors that are currently impacting his mental health.
At Holmusk, we are developing innovative natural language processing (NLP) models to extract and translate this extremely important information, both to support informed decision-making in a clinical setting and to aid in analysis for research.
In the scenario above, the value of NLP is evident. Without NLP, the ER doctor lacked the context needed to understand the stressor that precipitated the event. If NLP had been applied to the patient’s clinical notes, it would have revealed how he had been doing over time and provided perspective on socioenvironmental stressors and other factors affecting his mental wellbeing. Applying NLP to reveal stressors at specific time points along the patient’s journey increases the density and granularity of available data on the patient and enhances understanding of the patient's trajectory.
Structured data: The tip of the iceberg
Last week, my colleague Melissa gave an overview of a portion of the socioenvironmental stressors that are collected in a structured format via routine patient care and made available via Holmusk’s NeuroBlu Database. We are fortunate that this information is collected through these clinician observations and structured scales and assessments. However, much of the information recorded during a routine behavioral health visit does not correspond to a structured field within the EHR, meaning that clinicians capture the majority of valuable clinical context in free text clinical notes, or unstructured data.
Historically, there has not been an easy way to extract and use this information, which can be widely heterogeneous due to a lack of standardized language in the behavioral health field. The only way would be for a health professional were to read through each clinical note to make a determination about whether certain symptoms or socioenvironmental stressors were present. This is not a scalable solution, whether you’re a provider in a busy hospital setting or a researcher analyzing large datasets.
This is where our NLP models provide immense value. We utilize innovative natural language processing techniques to extract fit-for-purpose information in a structured format that is readily utilizable for research. Our natural language processing models, the first to be developed on behavioral health clinical data, comb through clinical notes, interpret the context, extract relevant information, and map it to structured variables in a common data model, to be made available via our NeuroBlu Database.
Each model is developed through combined expertise in data science and behavioral health conditions, all found within Holmusk’s staff. First, NLP experts from Holmusk’s data science team use clinically informed annotations to train, test, and validate each model. Once development and validation are complete, Holmusk’s team of trained clinicians validates the insights derived from each model. This process enables Holmusk to provide richer data than has ever before been available, while ensuring a high degree of clinical accuracy.
Our goal: Valuable information, available and usable
As we continuously expand and refine our NLP models, we are cognizant of how much information on socioenvironmental stressors is contained within clinical notes, and how much value these data would bring to players across the behavioral health field.
We are currently working on models to extract information on diverse aspects of behavioral health care delivery, including but not limited to symptoms, side effects, and socioenvironmental stressors such as family history. We envision a world in which there are research-ready variables to represent details about a patient’s environment that are currently not recorded in a structured way, from acute life changes such as loss of a loved one to more chronic stressors such as presence of trauma. Because these events and stressors can have serious impacts to a person’s behavioral health, it is important that we work toward a better, more structured process to identify and record them.
Our work highlighting socioenvironmental stressors, as well as the important role they play in behavioral health, was recently recognized at ISPOR. An analysis fueled by Holmusk’s NeuroBlu and led by my colleague Sherwin Kuah revealed not only that rates of suicidality among children have steadily increased between 2011 and 2019, but also took a look at which socioenvironmental factors may have played a role in this trend. Findings showed that family stressors were common, both for those who experienced suicidal symptoms and those who did not.
Ultimately, we are working to equip the behavioral health field with better understanding of psychiatric conditions, and we recognize that socioenvironmental stressors play a critical role in this understanding. With our NLP models, we unlock the previously untapped potential of clinical notes–while leveraging the appropriate clinical context–so that we can all learn as much as we can from the data that are already being captured as part of routine care delivery. By enabling understanding of the nuance involved in each patient’s story–and how their environments have contributed to these stories–we can advance the field toward its goals of developing targeted treatments and improving patient outcomes across the board.