Data Differentiators: Revealing New Information via Natural Language Processing
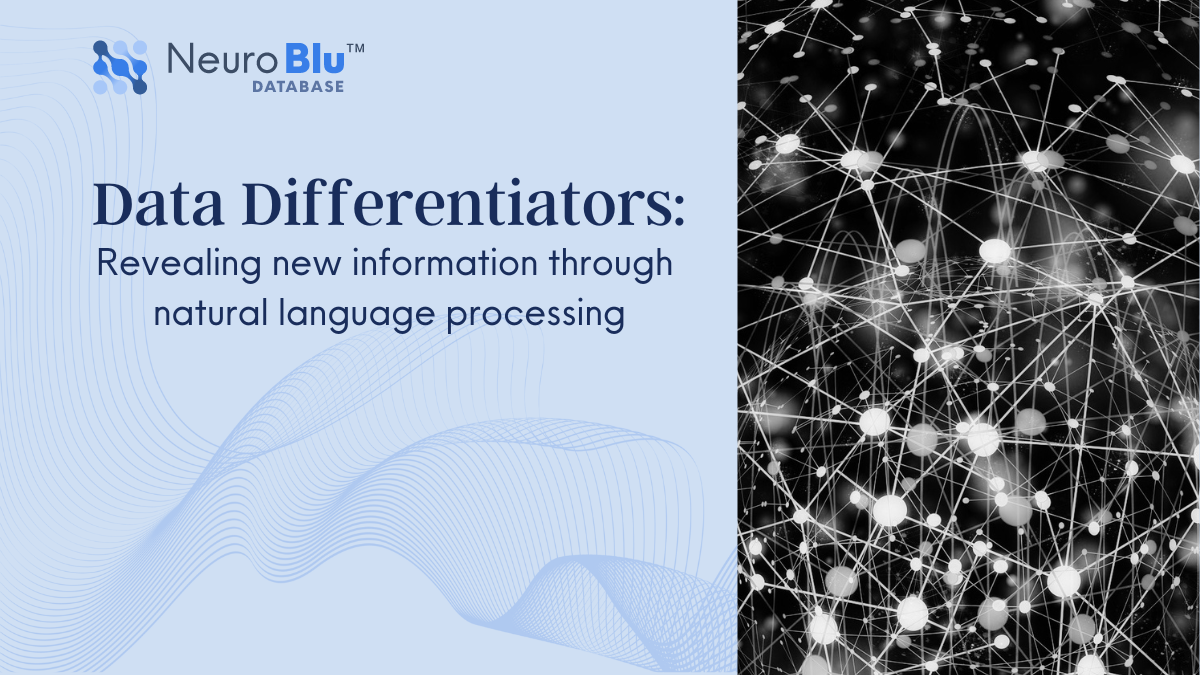
This blog post is a continuation of our Data Differentiators series, in which we’re sharing how the NeuroBlu Database stands out from other EHR-derived real-world data sources. For previous installments of the series, see here and here.
Retrospective studies in behavioral health lack granularity of the different clinical presentations that behavioral health disorders can take. At Holmusk, we have met this challenge and delved deeper into symptomology by developing a suite of first-in-kind natural language processing (NLP) models—the first created specifically for behavioral health.
Leveraging our natural language processing model has enabled us to differentiate our data even further; we now have unique, NLP-enriched data types not commonly found in other EHR-derived sources of real-world data. Our method has been published in peer-reviewed papers and is interpretable for clinicians and researchers.
We break down five cool facts about our NLP—and how our NLP-enriched data can help your research program:
- Typically, behavioral health providers document information about their patients in visit notes. This information, although rich and clinically meaningful, is unstructured and therefore has historically been unusable for research, unless it is translated in a time-consuming manual process. Our NLP transforms these unstructured data into structured, discrete variables, ensuring that all information available about a patient is available for research.
- Using our NLP models, we have created over 300 psychiatry-specific data labels, capturing a comprehensive picture of a patient’s symptoms that extends beyond the symptoms traditionally associated with the ICD-10 diagnosis code.
- This symptom granularity enables specific segmentation of patients to create robust cohorts for research. Some examples include: patients with major depressive disorder and suicidal intent, patients with schizophrenia and cognitive impairment, or patients with ADHD and executive function impairment.
- NLP helps to capture and extract symptoms at each patient visit rather than capturing this information at specific points in time. This allows researchers to track patients over time and ensures that a wide swath of patients are eligible for studies regardless of study design.
- One of our NLP models extracts information on external stressors, shedding light on important factors in a patient’s environment that may be impacting their health or disease presentation, such as finances, family, or social situations.
Want to see our NLP-enriched data for yourself? Get in touch.